Documents
Poster
ADT: ANTI-DEEPFAKE TRANSFORMER
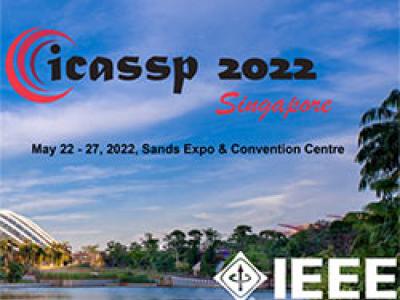
- Citation Author(s):
- Submitted by:
- ping wang
- Last updated:
- 5 May 2022 - 10:23am
- Document Type:
- Poster
- Document Year:
- 2022
- Event:
- Presenters:
- ping wang
- Paper Code:
- IFS-2.4
- Categories:
- Log in to post comments
Recently almost all the mainstream deepfake detection methods use Convolutional Neural Networks (CNN) as their backbone. However, due to the overreliance on local texture information, which is usually determined by forgery methods of training data, these CNN based methods cannot generalize well to unseen data. To get out of the predicament of prior methods, in this paper, we propose a novel transformer-based framework to model both global and local information and analyze anomalies of face images. In particular, we design attention leading module, multi-forensics module and variant residual connections for deepfake detection, and leverage token-level contrast loss for more detailed supervision. Experiments on almost all popular public deepfake datasets demonstrate that our method achieves state-of-the-art performance in cross-dataset evaluation and comparable performance in intra-dataset evaluation.