Documents
Presentation Slides
ADVERSARIAL EXAMPLE DETECTION BAYESIAN GAME
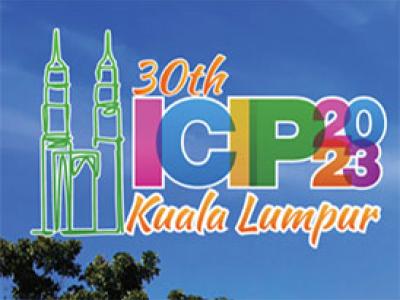
- DOI:
- 10.60864/ekqg-s358
- Citation Author(s):
- Submitted by:
- Frank Zeng
- Last updated:
- 17 November 2023 - 12:05pm
- Document Type:
- Presentation Slides
- Document Year:
- 2023
- Event:
- Presenters:
- Hui Zeng
- Paper Code:
- TA.L306.2
- Categories:
- Log in to post comments
Despite the increasing attack ability and transferability of adversarial examples (AE), their security, i.e., how unlikely they can be detected, has been ignored more or less. Without the ability to circumvent popular detectors, the chance that an AE successfully fools a deep neural network is slim. This paper gives a game theory analysis of the interplay between an AE attacker and an AE detection investigator. Taking the perspective of a third party, we introduce a game theory model to evaluate the ultimate performance when both the attacker and the investigator are aware of each other. Further, a Bayesian game is adopted to address the information asymmetry in practice. Solving the mixed-strategy Nash equilibrium of the game, both parties’ optimal strategies are obtained, and the security of AEs can be evaluated. We evaluate four popular attacks under a two-step test on ImageNet. The results may throw light on how a farsighted attacker or investigator will act in this adversarial environment. Our code is available at: https://github.com/zengh5/AED_BGame.