Documents
Poster
AEGIS-Net: Attention-Guided Multi-Level Feature Aggregation for Indoor Place Recognition
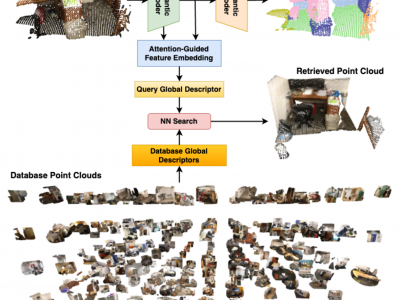
- DOI:
- 10.60864/hvtb-sh86
- Citation Author(s):
- Submitted by:
- Yuhang Ming
- Last updated:
- 6 June 2024 - 10:28am
- Document Type:
- Poster
- Document Year:
- 2024
- Event:
- Presenters:
- Yuhang Ming
- Paper Code:
- IVMSP-P15.3
- Categories:
- Log in to post comments
We present AEGIS-Net, a novel indoor place recognition model that takes in RGB point clouds and generates global place descriptors by aggregating lower-level color, geometry features and higher-level implicit semantic features. However, rather than simple feature concatenation, self-attention modules are employed to select the most important local features that best describe an indoor place. Our AEGIS-Net is made of a semantic encoder, a semantic decoder and an attention-guided feature embedding. The model is trained in a 2-stage process with the first stage focusing on an auxiliary semantic segmentation task and the second one on the place recognition task. We evaluate our AEGIS-Net on the ScanNetPR dataset and compare its performance with a pre-deep-learning feature-based method and five state-of-the- art deep-learning-based methods. Our AEGIS-Net achieves exceptional performance and outperforms all six methods.
Poster.pdf
