Documents
Presentation Slides
Aggregation Graph Neural Networks
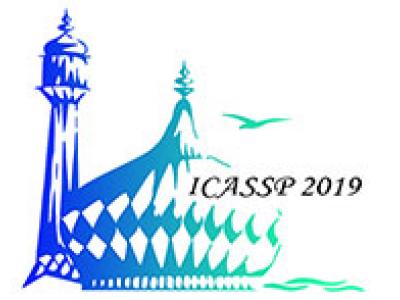
- Citation Author(s):
- Submitted by:
- Fernando Gama
- Last updated:
- 15 May 2019 - 2:34pm
- Document Type:
- Presentation Slides
- Document Year:
- 2019
- Event:
- Presenters:
- Fernando Gama
- Paper Code:
- 2457
- Categories:
- Keywords:
- Log in to post comments
Graph neural networks (GNNs) regularize classical neural networks by exploiting the underlying irregular structure supporting graph data, extending its application to broader data domains. The aggregation GNN presented here is a novel GNN that exploits the fact that the data collected at a single node by means of successive local exchanges with neighbors exhibits a regular structure. Thus, regular convolution and regular pooling yield an appropriately regularized GNN. To address some scalability issues that arise when collecting all the information at a single node, we propose a multi-node aggregation GNN that constructs regional features that are later aggregated into more global features and so on. We show superior performance in a source localization problem on synthetic graphs and on the authorship attribution problem.