Documents
Presentation Slides
AIR-TISSUE BOUNDARY SEGMENTATION IN REAL TIME MAGNETIC RESONANCE IMAGING VIDEO USING A CONVOLUTIONAL ENCODER-DECODER NETWORK
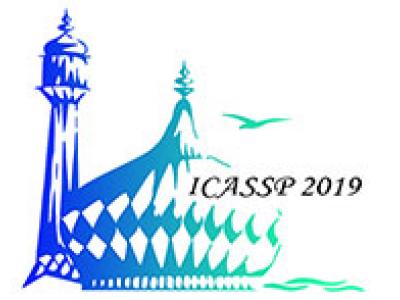
- Citation Author(s):
- Submitted by:
- Renuka Mannem
- Last updated:
- 8 May 2019 - 5:42am
- Document Type:
- Presentation Slides
- Document Year:
- 2019
- Event:
- Presenters:
- MANNEM RENUKA
- Paper Code:
- 4395
- Categories:
- Log in to post comments
In this paper, we propose a convolutional encoder-decoder network (CEDN) based approach for upper and lower Air-Tissue Boundary (ATB) segmentation within vocal tract in real-time magnetic resonance imaging (rtMRI) video frames. The output images from CEDN are processed using perimeter and moving average filters to generate smooth contours representing ATBs. Experiments are performed in both seen subject and unseen subject conditions to examine the generalizability of the CEDN based approach. The performance of the segmented ATBs is evaluated using Dynamic Time Warping distance between the ground truth contours and predicted contours. The proposed approach is compared with three baseline schemes - one grid-based unsupervised and two supervised schemes. Experiments with 5779 rtMRI images from four subjects show that the CEDN based approach performs better than the unsupervised baseline scheme by 8.5% for seen subjects case whereas it does better than the supervised baseline schemes only for lower ATB. For unseen subjects case, the proposed approach performs better than the supervised baseline schemes by 63.96%, 22.9% respectively whereas it performs worse than the unsupervised baseline scheme. However, the proposed approach outperforms the unsupervised baseline scheme when a minimum of 30 images from unseen subjects are used to adapt the trained CEDN model.