Documents
Poster
Analyzing Uncertainties in Speech Recognition Using Dropout
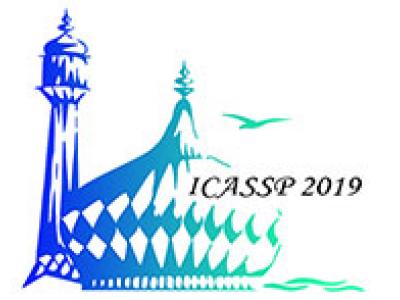
- Citation Author(s):
- Submitted by:
- Apoorv Vyas
- Last updated:
- 11 May 2019 - 8:55am
- Document Type:
- Poster
- Document Year:
- 2019
- Event:
- Presenters:
- Apoorv Vyas
- Paper Code:
- 4576
- Categories:
- Log in to post comments
The performance of Automatic Speech Recognition (ASR) systems is often measured using Word Error Rates (WER) which requires time-consuming and expensive manually transcribed data. In this paper, we use state-of-the-art ASR systems based on Deep Neural Networks (DNN) and propose a novel framework which uses ``Dropout'' at the test time to model uncertainty in prediction hypotheses. We systematically exploit this uncertainty to estimate WER without the need for explicit transcriptions. In addition, we show that the predictive uncertainty can also be used to accurately localize the errors made by the ASR system. We study the performance of our approach on Switchboard database where it predicts WER accurately within a range of 2.6% and 5.0% for HMM-DNN and Connectionist Temporal Classification (CTC) ASR systems, respectively.