Documents
Poster
ASSESSINGTHEIMPACTOFTHEDECEIVEDNONLOCALMEANSFILTERASA PREPROCESSINGSTAGEINACONVOLUTIONALNEURALNETWORKBASED APPROACHFORAGEESTIMATIONUSINGDIGITALHANDX-RAYIMAGES
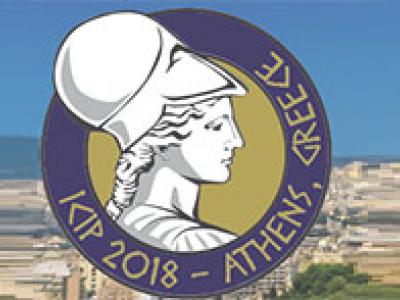
- Citation Author(s):
- Submitted by:
- Saul Calderon-R...
- Last updated:
- 6 October 2018 - 8:15am
- Document Type:
- Poster
- Document Year:
- 2018
- Event:
- Paper Code:
- 2714
- Categories:
- Log in to post comments
In this work we analyze the impact of denoising, contrast and edge enhancement using the Deceived Non Local Means (DNLM) filter in a Convolutional Neural Network (CNN) based approach for age estimation using digital X-ray images from hands. The DNLM filter contains two parameters which control edge enhancement and denoising. Increasing levels were tested to assess the impact of both contrast enhancement and denoising in the CNN based model regression accuracy. Results obtained showed that contrast enhancement was important for preprocessing in a CNN based approach, given a statistically significant 42% lower root mean squared error, with comparable to previous state of the art results, using larger publicly available dataset. The obtained results suggest that both image enhancement and denoising can significantly improve results in a CNN based model.