Documents
Poster
Automatic Defect Segmentation by Unsupervised Anomaly Learning
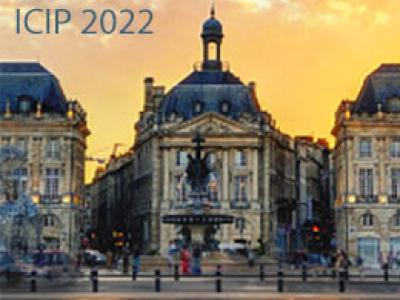
- Citation Author(s):
- Submitted by:
- Nati Ofir
- Last updated:
- 15 July 2022 - 1:17am
- Document Type:
- Poster
- Document Year:
- 2022
- Event:
- Presenters:
- Nati Ofir
- Paper Code:
- 1225
- Categories:
- Log in to post comments
This paper addresses the problem of defect segmentation in semiconductor manufacturing. The input of our segmentation is a scanning-electron-microscopy (SEM) image of the candidate defect region. We train a U-net shape network to segment defects using a dataset of clean background images. The samples of the training phase are produced automatically such that no manual labeling is required. To enrich the dataset of clean background samples, we apply defect implant augmentation. To that end, we apply a copy-and-paste of a random image patch in the clean specimen. To improve the robustness of the unlabeled data scenario, we train the features of the network with unsupervised learning methods and loss functions. Our experiments show that we succeed to segment real defects with high quality, even though our dataset contains no defect examples. Our approach performs accurately also on the problem of supervised and labeled defect segmentation.