Documents
Poster
Bayesian topology inference on partially known networks from input-output pairs
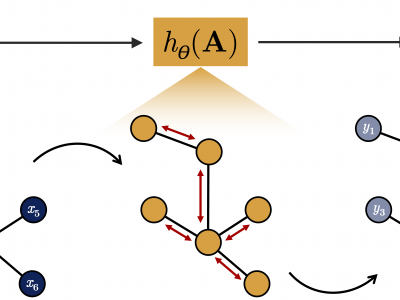
- DOI:
- 10.60864/vq4c-wd47
- Citation Author(s):
- Submitted by:
- Martin Sevilla
- Last updated:
- 3 April 2024 - 3:08pm
- Document Type:
- Poster
- Document Year:
- 2024
- Event:
- Presenters:
- Martín Sevilla
- Categories:
- Keywords:
- Log in to post comments
We propose a sampling algorithm to perform system identification from a set of input-output graph signal pairs. The dynamics of the systems we study are given by a partially known adjacency matrix and a generic parametric graph filter of unknown parameters. The methodology we employ is built upon the principles of annealed Langevin diffusion. This enables us to draw samples from the posterior distribution instead of following the classical approach of point estimation using maximum likelihood. We investigate how to harness the prior information inherent in a dataset of graphs of different sizes through the utilization of graph neural networks. We demonstrate, via numerical experiments involving both real-world and synthetic networks, that integrating prior knowledge into the estimation process enhances estimation performance.