Documents
Poster
CAUSALLY UNCOVERING BIAS IN VIDEO MICRO-EXPRESSION RECOGNITION
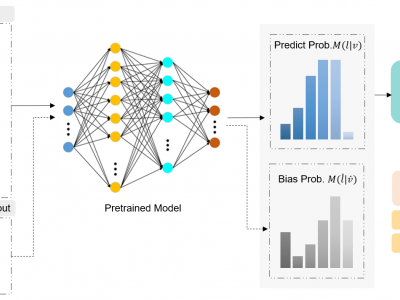
- DOI:
- 10.60864/tcpq-d423
- Citation Author(s):
- Submitted by:
- Tan Pei Sze
- Last updated:
- 6 June 2024 - 10:28am
- Document Type:
- Poster
- Document Year:
- 2024
- Event:
- Presenters:
- Tan Pei Sze
- Paper Code:
- MLSP-P37.2
- Categories:
- Keywords:
- Log in to post comments
Detecting microexpressions presents formidable challenges, primarily due to their fleeting nature and the limited diversity in existing datasets. Our studies find that these datasets exhibit a pronounced bias towards specific ethnicities and suffer from significant imbalances in terms of both class and gender representation among the samples. These disparities create fertile ground for various biases to permeate deep learning models, leading to skewed results and inadequate portrayal of specific demographic groups. Our research is driven by a compelling need to identify and rectify these biases within model architectures. To achieve this, we commence by constructing a causal graph that elucidates the intricate relationships between the model, input features, and training outcomes. This graphical representation forms the foundation for our analytical framework. Leveraging this causal framework, we conduct comprehensive case studies, employing counterfactuals as a diagnostic tool to unveil biases arising from dataset-induced class imbalances, gender inequalities, and variations in facial action units. Our final step involves a highly efficient counterfactual debiasing process, eliminating the necessity for additional data collection or model retraining. Our results showcase superior performance compared to state-of-the-art methods across the CASME II, SAMM, and SMIC datasets.