Documents
Presentation Slides
A-CCNN: Adaptive CCNN for Density Estimation and Crowd Counting
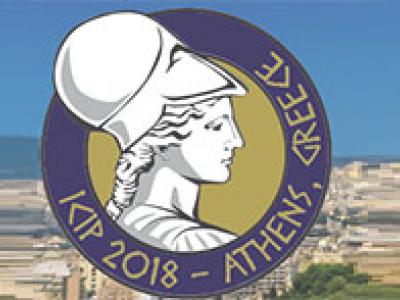
- Citation Author(s):
- Submitted by:
- Saeed Amirgholipour
- Last updated:
- 10 October 2018 - 7:26am
- Document Type:
- Presentation Slides
- Document Year:
- 2018
- Event:
- Presenters:
- Saeed Amirgholipour
- Paper Code:
- 2131
- Categories:
- Keywords:
- Log in to post comments
Crowd counting, for estimating the number of people in a crowd using vision-based computer techniques, has attracted much interest in the research community. Although many attempts have been reported, real-world problems, such as huge variation in subjects’ sizes in images and serious occlusion among people, make it still a challenging problem. In this paper, we propose an Adaptive Counting Convolutional Neural Network (A-CCNN) and consider the scale variation of objects in a frame adaptively so as to improve the accuracy of counting. Our method takes advantages of contextual information to provide more accurate and adaptive density maps and crowd counting in a scene. Extensively experimental evaluation is conducted using different benchmark datasets for object-counting and shows that the proposed approach is effective and outperforms state-of-the-art approaches.
ICIP2018.pptx
