Documents
Poster
CHANNEL-SPATIAL TRANSFORMER FOR EFFICIENT IMAGE SUPER-RESOLUTION
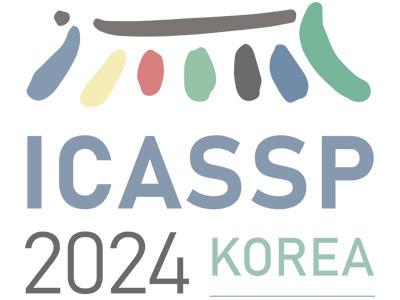
- DOI:
- 10.60864/0z9t-za83
- Citation Author(s):
- Submitted by:
- Jiuqiang Li
- Last updated:
- 6 June 2024 - 10:23am
- Document Type:
- Poster
- Document Year:
- 2024
- Event:
- Categories:
- Log in to post comments
Transformer has achieved remarkable success in low-level visual tasks, including image super-resolution (SR), owing to its ability to establish global dependencies through self-attention mechanism. However, existing methods overlook the mutual influence and promotion between the channel and spatial dimensions. The feed-forward network (FFN) in the transformer architecture introduces redundant information in the channel during feature extraction, hindering feature representation capability and neglecting spatial information modeling. To address these limitations, we propose a Channel-Spatial Transformer (CST) that combines self-attention from both channel and spatial perspectives to extract more reliable deep features. Specifically, we carefully design Channel Self-Attention Module (CSAM) and Spatial Self-Attention Module (SSAM) within the transformer architecture, which compute attention along the channel dimension and within spatial windows, respectively. Additionally, we introduce Channel-Spatial Feed-forward Network (CSFN), which addresses the limitations of traditional FFN in the channel and spatial domains by employing global average pooling and depth-wise convolution parallelly. Extensive experiments demonstrate superior performance of CST compared to existing methods.
poster_1446.pdf
