Documents
Presentation Slides
Characterizing the adversarial vulnerability of speech self-supervised learning
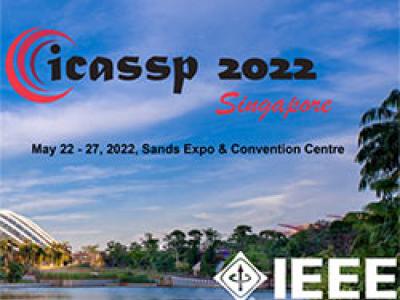
- Citation Author(s):
- Submitted by:
- Haibin Wu
- Last updated:
- 4 May 2022 - 11:39pm
- Document Type:
- Presentation Slides
- Document Year:
- 2022
- Event:
- Presenters:
- Haibin Wu
- Paper Code:
- MLSP-3.1
- Categories:
- Log in to post comments
A leaderboard named Speech processing Universal PERformance Benchmark (SUPERB), which aims at benchmarking the performance of a shared self-supervised learning (SSL) speech model across various downstream speech tasks with minimal modification of architectures and a small amount of data, has fueled the research for speech representation learning. The SUPERB demonstrates speech SSL upstream models improve the performance of various downstream tasks through just minimal adaptation. As the paradigm of the self-supervised learning upstream model followed by downstream tasks arouses more attention in the speech community, characterizing the adversarial robustness of such paradigm is of high priority. In this paper, we make the first attempt to investigate the adversarial vulnerability of such paradigm under the attacks from both zero-knowledge adversaries and limited-knowledge adversaries. The experimental results illustrate that the paradigm proposed by SUPERB is seriously vulnerable to limited-knowledge adversaries, and the attacks generated by zero-knowledge adversaries are with transferability. The XAB test verifies the imperceptibility of crafted adversarial attacks.