Documents
Presentation Slides
Class-specific Coders for Hyper-spectral Image Classification
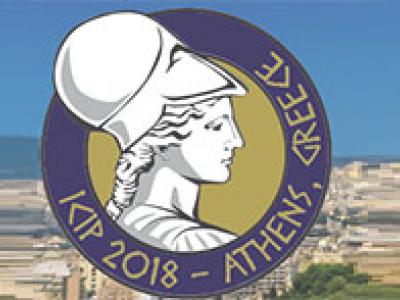
- Citation Author(s):
- Submitted by:
- Omkar Gune
- Last updated:
- 5 October 2018 - 2:35am
- Document Type:
- Presentation Slides
- Document Year:
- 2018
- Event:
- Presenters:
- Subhasis Chaudhuri
- Paper Code:
- ICIP18001
- Categories:
- Log in to post comments
In this paper, we introduce the paradigm of class specific
coders (CSC) for classification of hyperspectral images
(HSI). Apparently, CSC are defined as a set of distinct
encoder-decoder (henceforth called a coder) networks where
a given coder is trained on the samples of a particular class.
In contrast to auto-encoders (AE) which learn an identity
mapping of data in an unsupervised fashion, the CSC model,
on the other hand, learns re-constructive mappings for all
possible pairs of training samples for each class in separate
coders. Further, for reducing redundancy, it is ensured that
the latent space dimensions of the coders are orthogonal to
each other. Once the CSC are trained, we introduce a feature
encoding by concatenating the latent representations of all
the class-specific coders. Experimental results obtained on
the benchmark Botswana and Indian Pines datasets ensure
that the CSC model outperforms a number of AE variants
significantly.