Documents
Research Manuscript
Classifying Non-Individual Head-Related Transfer Functions with A Computational Auditory Model: Calibration And Metrics
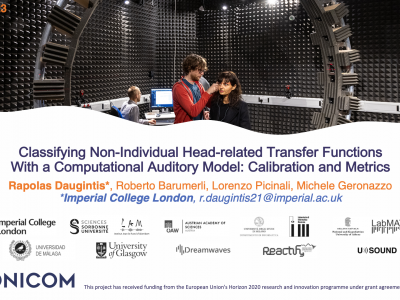
- DOI:
- 10.60864/fjez-9845
- Citation Author(s):
- Submitted by:
- Rapolas Daugintis
- Last updated:
- 17 November 2023 - 12:07pm
- Document Type:
- Research Manuscript
- Document Year:
- 2023
- Event:
- Presenters:
- Rapolas Daugintis
- Paper Code:
- 4813
- Categories:
- Log in to post comments
This study explores the use of a multi-feature Bayesian auditory sound localisation model to classify non-individual head-related transfer functions (HRTFs). Based on predicted sound localisation performance, these are grouped into ‘good’ and ‘bad’, and the ‘best’/‘worst’ is selected from each category. Firstly, we present a greedy algorithm for automated individual calibration of the model based on the individual sound localisation data. We then discuss data analysis of predicted directional localisation errors and present an algorithm for categorising the HRTFs based on the localisation error distributions within a limited range of directions in front of the listener. Finally, we discuss the validity of the classification algorithm when using averaged instead of individual model parameters. This analysis of auditory modelling results aims to provide a perceptual foundation for automated HRTF personalisation techniques for an improved experience of binaural spatial audio technologies.