Documents
Poster
Complex-Valued Vs. Real-Valued Neural Networks for Classification Perspectives: An Example on Non-Circular Data
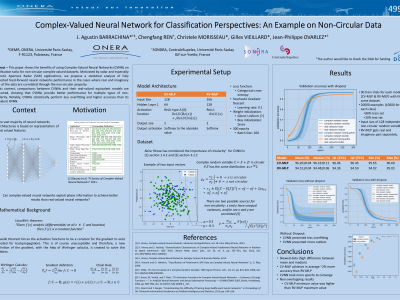
- Citation Author(s):
- Submitted by:
- Agusstin Barrachina
- Last updated:
- 23 June 2021 - 7:54am
- Document Type:
- Poster
- Document Year:
- 2021
- Event:
- Presenters:
- J Agustin BARRACHINA
- Paper Code:
- MLSP-9.6
- Categories:
- Log in to post comments
This paper shows the benefits of using Complex-Valued Neural Network (CVNN) on classification tasks for non-circular complex-valued datasets. Motivated by radar and especially Synthetic Aperture Radar (SAR) applications, we propose a statistical analysis of fully connected feed-forward neural networks performance in the cases where real and imaginary parts of the data are correlated through the non-circular property. In this context, comparisons between CVNNs and their real-valued equivalent models are conducted, showing that CVNNs provide better performance for multiple types of non-circularity. Notably, CVNNs statistically perform less overfitting, higher accuracy and provide shorter confidence intervals than its equivalent Real-Valued Neural Network (RVNN).