Documents
Presentation Slides
On Convergence of Projected Gradient Descent for Minimizing a Large Scale Quadratic over the Unit Sphere
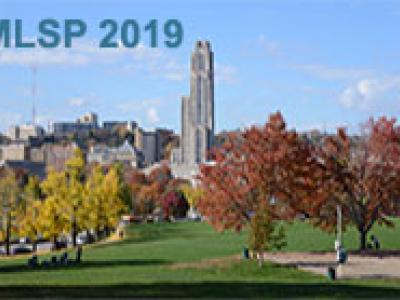
- Citation Author(s):
- Submitted by:
- Trung Vu
- Last updated:
- 26 October 2019 - 2:40pm
- Document Type:
- Presentation Slides
- Document Year:
- 2019
- Event:
- Presenters:
- Trung Vu
- Paper Code:
- MLSP-L6.2
- Categories:
- Log in to post comments
Unit sphere-constrained quadratic optimization has been studied extensively over the past decades. While state-of-art algorithms for solving this problem often rely on relaxation or approximation techniques, there has been little research into scalable first-order methods that tackle the problem in its original form. These first-order methods are often more well-suited for the big data setting. In this paper, we provide a novel analysis of the simple projected gradient descent method for minimizing a quadratic over a sphere. When the gradient step size is sufficiently small, we show that convergence is locally linear and provide a closed-form expression for the rate. Moreover, a careful selection of the step size can stimulate convergence to the global solution while preventing convergence to local minima.
MLSP2019.pdf
