Documents
Poster
CONVOLUTIONAL-SPARSE-CODED DYNAMIC MODE DECOMPOSITION AND ITS APPLICATION TO RIVER STATE ESTIMATION
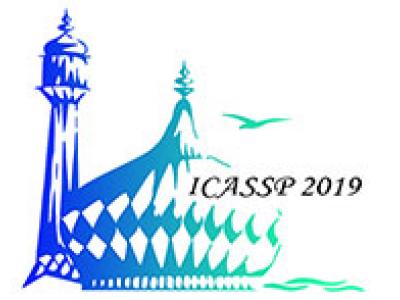
- Citation Author(s):
- Submitted by:
- Yuhei Kaneko
- Last updated:
- 10 May 2019 - 10:09am
- Document Type:
- Poster
- Document Year:
- 2019
- Event:
- Presenters:
- Yuhei Kaneko
- Paper Code:
- IVMSP-P2.7
- Categories:
- Log in to post comments
This work proposes convolutional-sparse-coded dynamic mode decomposition (CSC-DMD) by unifying extended dynamic mode de-
composition (EDMD) and convolutional sparse coding. EDMD is a data-driven method of analysis used to describe a nonlinear dynamical system with a linear time-evolution equation. Compared with existing EDMD methods, CSC-DMD has the advantage of reflecting the spatial structure of a target. As an example, the proposed method is applied to river bed shape estimation from the water surface observation. This estimation problem is reduced to sparsity-aware signal restoration with a hard constraint given by the CSC-DMD prediction, where the algorithm is derived by the primal-dual splitting method. A time series set of water surface and bed shape measured through an experimental river setup is used to train and test the system. From the result, the efficacy of the proposed method is verified.