Documents
Poster
Correlation-Aware Attention Branch Network Using Multi-Modal Data For Deterioration Level Estimation Of Infrastructures
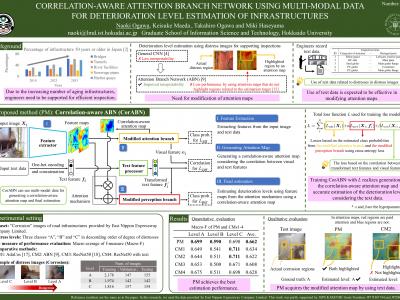
- Citation Author(s):
- Submitted by:
- Naoki Ogawa
- Last updated:
- 25 September 2021 - 5:00am
- Document Type:
- Poster
- Document Year:
- 2021
- Event:
- Presenters:
- Naoki Ogawa
- Paper Code:
- 3037
- Categories:
- Log in to post comments
This paper presents a correlation-aware attention branch network (CorABN) using multi-modal data for deterioration level estimation of infrastructures. CorABN can collaboratively use visual features from distress images and text features from text data recorded at the inspection to improve the estimation accuracy of deterioration levels. Specifically, by maximizing correlation between the visual and text features that provide useful information for the deterioration level estimation, a correlation-aware attention map can be generated. Besides, the text features are also utilized in the final estimation along with visual features improved by the attention mechanism to achieve higher estimation accuracy. With the losses based on both the estimation accuracy and the correlation, CorABN can train the entire model in an end-to-end manner. Experiments using distress images and their corresponding text data show the effectiveness of the proposed method.