Documents
Poster
CROSS BRANCH FEATURE FUSION DECODER FOR CONSISTENCY REGULARIZATION-BASED SEMI-SUPERVISED CHANGE DETECTION
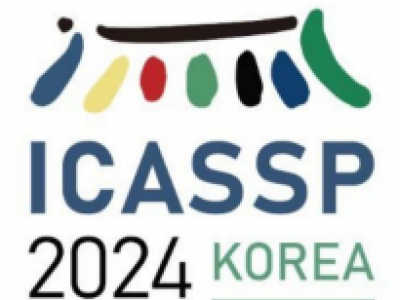
- DOI:
- 10.60864/cyf0-3t74
- Citation Author(s):
- Submitted by:
- Qi'ao Xu
- Last updated:
- 6 June 2024 - 10:32am
- Document Type:
- Poster
- Document Year:
- 2024
- Event:
- Paper Code:
- SPTM-P4.1
- Categories:
- Log in to post comments
Semi-supervised change detection (SSCD) utilizes partially labeled data and a large amount of unlabeled data to detect changes. However, the transformer-based SSCD network does not perform as well as the convolution-based SSCD network due to the lack of labeled data. To overcome this limitation, we introduce a new decoder called Cross Branch Feature Fusion (CBFF), which combines the strengths of both local convolutional branch and global transformer branch. The convolutional branch is easy to learn and can produce high-quality features with a small amount of labeled data. The transformer branch, on the other hand, can extract global context features but is hard to learn without a lot of labeled data. Using CBFF, we build our SSCD model based on a strong-to-weak consistency strategy. Through comprehensive experiments on WHU-CD and LEVIR-CD datasets, we have demonstrated the superiority of our method over seven state-of-the-art SSCD methods.