Documents
Poster
DANET: DEPTH-AWARE NETWORK FOR CROWD COUNTING
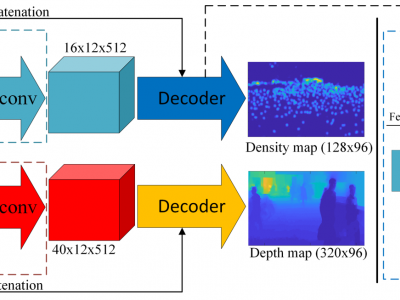
- Citation Author(s):
- Submitted by:
- LAI CHEN TUNG
- Last updated:
- 20 September 2019 - 10:06am
- Document Type:
- Poster
- Document Year:
- 2019
- Event:
- Presenters:
- Chen Tung Lai
- Paper Code:
- #2566
- Categories:
- Log in to post comments
Image-based people counting is a challenging work due to the large scale variation problem caused by the diversity of distance between the camera and the person, especially in the congested scenes. To handle this problem, the previous methods focus on building complicated models and rely on labeling the sophisticated density maps to learn the scale variation implicitly. It is often time-consuming in data pre-processing and difficult to train these deep models due to the lack of training data. In this paper, we thus propose an alternative and novel way for crowd counting which handles the scale variation problem by leveraging the auxiliary depth estimation dataset. Using separated crowd and depth datasets, we train a unified network for two tasks- crowd density map estimation and depth estimation- at the same time. By introducing the auxiliary depth estimation task, we prove that the scale problem caused by distance can be well solved and the labeling cost can be reduced. The efficacy of our method is demonstrated in the extensive experiments by multiple evaluation criteria.