Documents
Presentation Slides
A Data-driven Cognitive Salience Model for Objective Perceptual Audio Quality Assessment
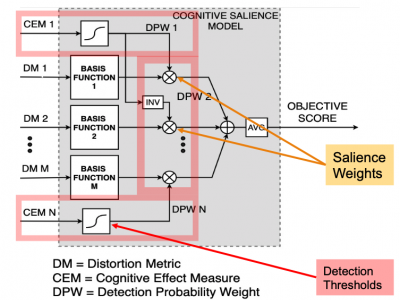
- Citation Author(s):
- Submitted by:
- Pablo Delgado
- Last updated:
- 13 May 2022 - 5:43am
- Document Type:
- Presentation Slides
- Document Year:
- 2022
- Event:
- Presenters:
- Pablo Manuel Delgado
- Paper Code:
- 1857
- Categories:
- Log in to post comments
Objective audio quality assessment systems often use perceptual models to predict the subjective quality scores of processed signals, as reported in listening tests. Most systems map different metrics of perceived degradation into a single quality score predicting subjective quality. This requires a quality mapping stage that is informed by real listening test data using statistical learning (\iec a data-driven approach) with distortion metrics as input features. However, the amount of reliable training data is limited in practice, and usually not sufficient for a comprehensive training of large learning models. Models of cognitive effects in objective systems can, however, improve the learning model. Specifically, considering the salience of certain distortion types, they provide additional features to the mapping stage that improve the learning process, especially for limited amounts of training data. We propose a novel data-driven salience model that informs the quality mapping stage by explicitly estimating the cognitive/degradation metric interactions using a salience measure. Systems incorporating the novel salience model are shown to outperform equivalent systems that only use statistical learning to combine cognitive and degradation metrics, as well as other well-known measurement systems, for a representative validation dataset.