Documents
Poster
DATA-SCARCE CONDITION MODELING REQUIRES MODEL-BASED PRIOR REGULARIZATION
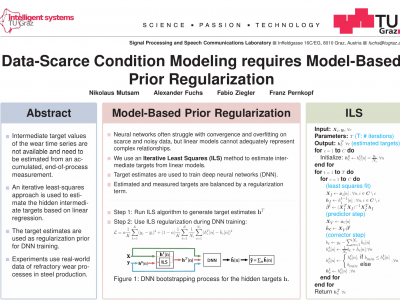
- Citation Author(s):
- Submitted by:
- Alexander Fuchs
- Last updated:
- 11 April 2024 - 12:12pm
- Document Type:
- Poster
- Document Year:
- 2024
- Event:
- Presenters:
- Alexander Fuchs
- Paper Code:
- MLSP-P30
- Categories:
- Keywords:
- Log in to post comments
In the metallurgical industry, taking measurements during production can be infeasible or undesired, and only the terminated process can be measured. This poses problems for regression models, as the intermediate target values for a time series are hidden in the accumulated end-of-process measurement. The lack of data quality and quantity also often limits the modeling to linear estimators, as neural networks struggle to converge and/or overfit on scarce noisy data. In this paper, we present a model-based prior for regularized training of neural networks for refractory wear modeling to handle scarce datasets with partially hidden targets. We use an iterative least-squares approach for mutual estimation of intermediate target values, which are then further used as a regularization prior for neural network training. We provide experimental results for refractory wear modeling of two distinct steel processing vessel types. Our results show substantial improvements in wear prediction performance.