Documents
Poster
Deep and Ordinal Ensemble Learning for Human Age Estimation From Facial Images
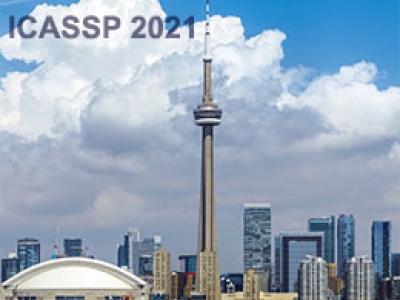
- Citation Author(s):
- Submitted by:
- Jiu-Cheng Xie
- Last updated:
- 21 June 2021 - 11:10pm
- Document Type:
- Poster
- Document Year:
- 2021
- Event:
- Presenters:
- Jiu-Cheng Xie
- Categories:
- Log in to post comments
Some recent work treats age estimation as an ordinal ranking task and decomposes it into multiple binary classifications. However, a theoretical defect lies in this type of methods: the ignorance of possible contradictions in individual ranking results. In this paper, we partially embrace the decomposition idea and propose the Deep and Ordinal Ensemble Learning with Two Groups Classification (DOEL 2groups ) for age prediction. An important advantage of our approach is that it theoretically allows the prediction even when the contradictory cases occur. The proposed method is characterized by a deep and ordinal ensemble and a two-stage aggregation strategy. Specifically, we first set up the ensemble based on Convolutional Neural Network (CNN) techniques, while the ordinal relationship is implicitly constructed among its base learners. Each base learner will classify the target face into one of two specific age groups. After achieving probability predictions of different age groups, then we make aggregation by transforming them into counting value distributions of whole age classes and getting the final age estimation from their votes. Moreover, to further improve the estimation performance, we suggest to regard the age class at the boundary of original two age groups as another age group and this modified version is named the Deep and Ordinal Ensemble Learning with Three Groups Classification (DOEL 3groups ). Effectiveness of this new grouping scheme is validated in theory and practice. Finally, we evaluate the proposed two ensemble methods on controlled and wild aging databases, and both of them produce competitive results. Note that the DOEL 3groups shows the state-of-the-art performance in most cases.
DOEL_poster.pdf
