Documents
Presentation Slides
Deep Feature Embedding Learning for Person Re-Identification Using Lifted Structured Loss
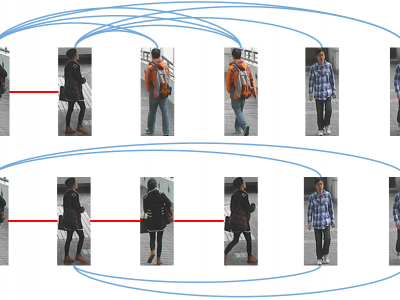
- Citation Author(s):
- Submitted by:
- Cheolkon Jung
- Last updated:
- 20 April 2018 - 5:23am
- Document Type:
- Presentation Slides
- Document Year:
- 2018
- Event:
- Presenters:
- Cheolkon Jung
- Categories:
- Keywords:
- Log in to post comments
In this paper, we propose deep feature embedding learning for person re-identification (re-id) using lifted structured loss. Although triplet loss has been commonly used in deep neural networks for person re-id, the triplet loss-based framework is not effective in fully using the batch information. Thus, it needs to choose hard negative samples manually that is very time-consuming. To address this problem, we adopt lifted structured loss for deep neural networks that makes the network learn better feature embedding by minimizing intra-class variation and maximizing inter-class variation. Extensive experiments on CUHK03, CUHK01 and VIPeR datasets demonstrate the superior performance of the proposed method over state-of-the-arts in terms of the cumulative match curve (CMC) metric.