Documents
Poster
Deep Fusion of Multi-Object Densities Using Transformer
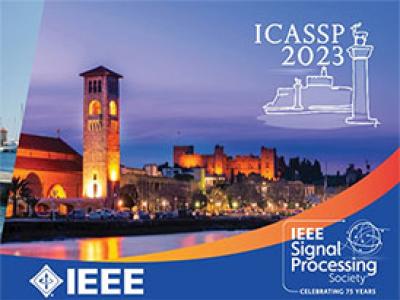
- DOI:
- 10.60864/2mkp-7s54
- Citation Author(s):
- Submitted by:
- Yuxuan Xia
- Last updated:
- 17 November 2023 - 12:07pm
- Document Type:
- Poster
- Document Year:
- 2023
- Event:
- Presenters:
- Yuxuan Xia
- Paper Code:
- 268
- Categories:
- Log in to post comments
The fusion of multiple probability densities has important applications in many fields, including, for example, multi-sensor signal pro- cessing, robotics, and smart environments. In this paper, we demonstrate that deep learning-based methods can be used to fuse multi-object densities. Given a scenario with several sensors with possibly different field-of-views, tracking is performed locally in each sensor by a tracker, which produces random finite set multi-object densities. To fuse outputs from different trackers, we adapt a recently proposed transformer-based multi-object tracker, where the fusion result is a global multi-object density, describing the set of all alive objects at the current time. We compare the performance of the transformer-based fusion method with a well-performing model-based Bayesian fusion method in several simulated scenarios with different parameter settings using synthetic data. The simulation results show that the transformer-based fusion method outperforms the model-based Bayesian method in our experimental scenarios. The code is available at https://github.com/Lechili/DeepFusion.