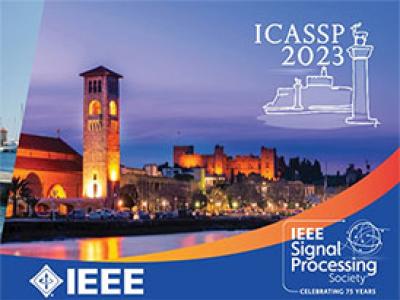
The fusion of multiple probability densities has important applications in many fields, including, for example, multi-sensor signal pro- cessing, robotics, and smart environments. In this paper, we demonstrate that deep learning-based methods can be used to fuse multi-object densities. Given a scenario with several sensors with possibly different field-of-views, tracking is performed locally in each sensor by a tracker, which produces random finite set multi-object densities.
- Categories:
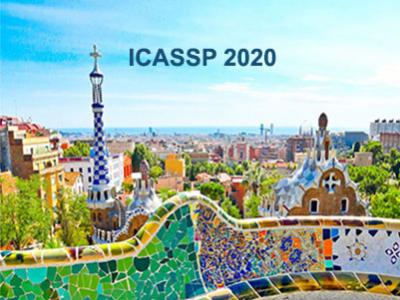
- Read more about Performance Bounds for Displaced Sensor Automotive Radar Imaging
- Log in to post comments
In automotive radar imaging, displaced sensors offer improvement in localization accuracy by jointly processing the data acquired from multiple radar units, each of which may have limited individual resources. In this paper, we derive performance bounds on the estimation error of target parameters processed by displaced sensors that correspond to several independent radars mounted at different locations on the same vehicle. Unlike previous studies, we do not assume a very accurate time synchronization among the sensors.
- Categories:
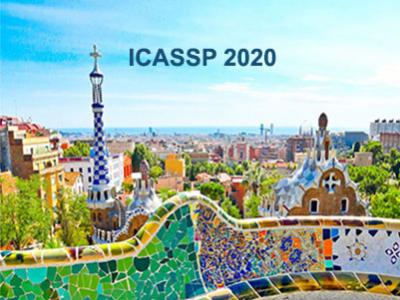
- Read more about Performance Bounds for Displaced Sensor Automotive Radar Imaging
- Log in to post comments
In automotive radar imaging, displaced sensors offer improvement in localization accuracy by jointly processing the data acquired from multiple radar units, each of which may have limited individual resources. In this paper, we derive performance bounds on the estimation error of target parameters processed by displaced sensors that correspond to several independent radars mounted at different locations on the same vehicle. Unlike previous studies, we do not assume a very accurate time synchronization among the sensors.
- Categories:
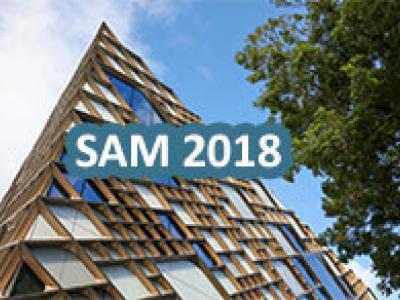
- Read more about Performance Analysis of Multi-hop Relaying Cooperation for Internet-of-things System
- Log in to post comments
- Categories:
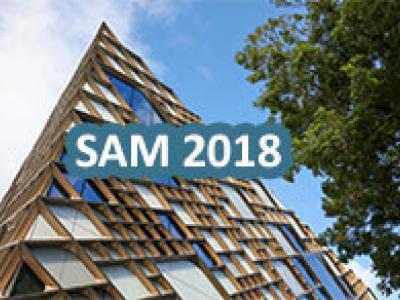
- Read more about A Neyman-Pearson Type Sensor Censoring Scheme for Compressive Distributed Sparse Signal Recovery
- Log in to post comments
To strike a balance between energy efficiency and data quality control, this paper proposes a Neyman-Pearson type sensor censoring scheme for distributed sparse signal recovery via compressive-sensing based on wireless sensor networks. In the proposed approach, each sensor node employs a sparse sensing vector with known support for data compression, meanwhile enabling making local inference about the unknown support of the sparse signal vector of interest.
- Categories:
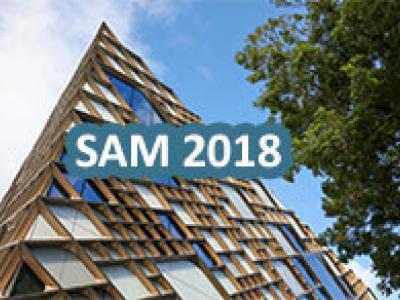
- Read more about Tracking Multiple Evolving Threats With Cluttered Surveillance Observations
- Log in to post comments
Many threats in the form of human actions (terrorist attacks, military actions, etc.) can be stochastically modeled by someone with relevant expert knowledge. In this work, a threat is taken to be a modeled sequence of actions that evolve over time and culminate at some ultimate goal. A model would be a hypothesis as to how a threat would develop, and what kind of observable evidence it would produce along the way. This modeling method allows us to attempt detection using the preliminary evidence of a threat.
SAM_Paper.pdf

- Categories:
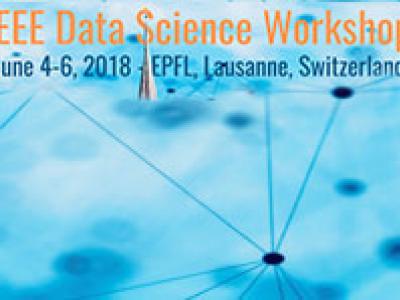
- Read more about Nonparametric Distributed Detection Using One-Sample Anderson-Darling Test and p-value Fusion
- Log in to post comments
In this paper a method for distributed detection for scenarios when there is no explicit knowledge of the probability models associated with the hypotheses is proposed. The underlying distributions are accurately learned from the data by bootstrapping. We propose using a nonparametric one-sample Anderson-Darling test locally at each sensor. The one-sample version of the test gives superior performance in comparison to the two-sample alternative. The local decision statistics, in particular p-values are then sent to a fusion center to make the final decision.
- Categories:
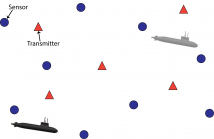
- Read more about Scheduling of Multistatic Sonobuoy Fields using Multi-Objective Optimization
- Log in to post comments
Sonobuoy fields, comprising a network of transmitters and receivers, are commonly deployed to find and track underwater targets. For a given environment and sonobuoy field layout, the performance of such a field depends on the scheduling, that is, deciding which source should transmit, and which from a library of available waveforms should be transmitted at any given time. In this paper, we propose a novel scheduling framework based on multi-objective optimization. Specifically, we pose the two tasks of the sonobuoy field—tracking and searching—as separate, competing, objective functions.
Sonar_Slides.pdf

- Categories:
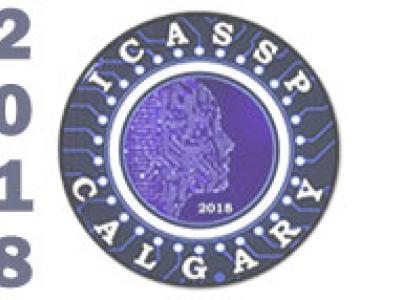
- Read more about A hybrid dictionary approach for distributed kernel adaptive filtering in diffusion networks
- Log in to post comments
ANTposter.pdf

- Categories:

- Read more about PARAMETER ESTIMATION FROM HETEROGENEOUS/MULTIMODAL DATA SETS
- Log in to post comments
Optimal parameter estimation requires simultaneous processing of all available measurements.
The complexity of this task may become too large when measurements from two or more multimodal sensor networks are avaliable.
In such cases, fusion of estimates obtained from each data set separately may be practical.
In this paper, we derive the optimal linear combination of the possibly non-linear estimators, and propose sub-optimal weightings.
We analyze the asymptotic performance gain of the first suboptimal approach with respect to the individual optimal estimates.
- Categories: