Documents
Poster
DEEP HIDDEN ANALYSIS: A STATISTICAL FRAMEWORK TO PRUNE FEATURE MAPS
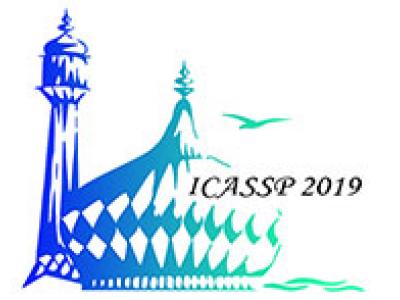
- Citation Author(s):
- Submitted by:
- Arshdeep Singh
- Last updated:
- 7 May 2019 - 1:19am
- Document Type:
- Poster
- Document Year:
- 2019
- Event:
- Presenters:
- Arshdeep Singh
- Paper Code:
- AASP-P13
- Categories:
- Log in to post comments
In this paper, we propose a statistical framework to prune feature maps in 1-D deep convolutional networks. SoundNet is a pre-trained deep convolutional network that accepts raw audio samples as input. The feature maps generated at various layers of SoundNet have redundancy, which can be identified by statistical analysis. These redundant feature maps can be pruned from the network with a very minor reduction in the capability of the network. The advantage of pruning feature maps, is that computational complexity can be reduced in the context of using an ensemble of classifiers on the layers of SoundNet. Our experiments on acoustic scene classification demonstrate that ignoring 89% of feature maps reduces the performance by less than 3% with 18% reduction in computational complexity.