Documents
Poster
DEEP SPEAKER REPRESENTATION USING ORTHOGONAL DECOMPOSITION AND RECOMBINATION FOR SPEAKER VERIFICATION
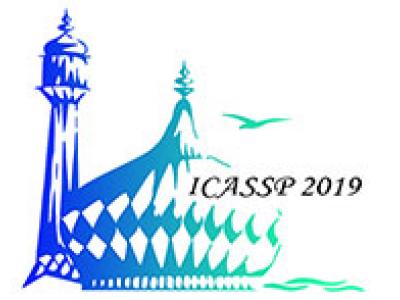
- Citation Author(s):
- Submitted by:
- Insoo Kim
- Last updated:
- 13 May 2019 - 2:29am
- Document Type:
- Poster
- Document Year:
- 2019
- Event:
- Presenters:
- Insoo Kim
- Paper Code:
- 1161
- Categories:
- Log in to post comments
Speech signal contains intrinsic and extrinsic variations such as accent, emotion, dialect, phoneme, speaking manner, noise, music, and reverberation. Some of these variations are unnecessary and are unspecified factors of variation. These factors lead to increased variability in speaker representation. In this paper, we assume that unspecified factors of variation exist in speaker representations, and we attempt to minimize variability in speaker representation. The key idea is that a primal speaker representation can be decomposed into orthogonal vectors and these vectors are recombined by using deep neural networks (DNN) to reduce speaker representation variability, yielding performance improvement for speaker verification (SV). The experimental results show that our proposed approach produces a relative equal error rate (EER) reduction of 47.1% compared to the use of the same convolutional neural network (CNN) architecture on the VoxCeleb dataset. Furthermore, our proposed method provides significant improvement for short utterances.