Documents
Poster
DEPTH REMOVAL DISTILLATION FOR RGB-D SEMANTIC SEGMENTATION
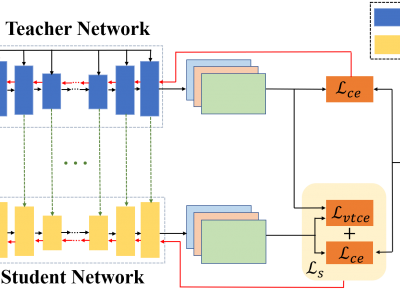
- Citation Author(s):
- Submitted by:
- Tiyu Fang
- Last updated:
- 6 May 2022 - 4:34am
- Document Type:
- Poster
- Document Year:
- 2022
- Event:
- Presenters:
- Tiyu Fang
- Paper Code:
- IVMSP-27.6
- Categories:
- Log in to post comments
RGB-D semantic segmentation is attracting wide attention due to its better performance than conventional RGB methods. However, most of RGB-D semantic segmentation methods need to acquire the real depth information for segmenting RGB images effectively. Therefore, it is extremely challenging to take full advantage of RGB-D semantic segmentation methods for segmenting RGB images without the depth input. To address this challenge, a general depth removal distillation method is proposed to remove depth dependence from RGB-D semantic segmentation model by knowledge distillation, which can be employed to any CNN-based segmentation network structure. Specifically, a depth-aware convolution is adopted to construct the teacher network for getting sufficient knowledge from RGB-D images. Then according to the structure consistency between depth-aware convolution and general convolution, the teacher network is used to transfer the learned knowledge to the student network with general convolutions by sharing parameters. Next, the student network makes up for the lack of depth in manner of learning by RGB images. Meantime, a Variable Temperature Cross Entropy (VTCE) loss function is proposed to further increase the accuracy of the student model by soft target distillation. Extensive experiments on NYUv2 and SUN RGB-D datasets demonstrate the superiority of our proposed approach.