Documents
Presentation Slides
DIFFUSION-BASED SPEECH ENHANCEMENT IN MATCHED AND MISMATCHED CONDITIONS USING A HEUN-BASED SAMPLER
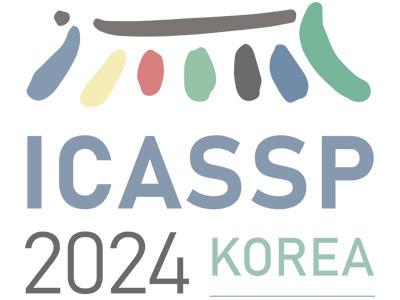
- Citation Author(s):
- Submitted by:
- Philippe Gonzalez
- Last updated:
- 15 April 2024 - 4:35am
- Document Type:
- Presentation Slides
- Event:
- Presenters:
- Philippe Gonzalez
- Paper Code:
- SLP-L1.1
- Categories:
- Log in to post comments
Diffusion models are a new class of generative models that have recently been applied to speech enhancement successfully. Previous works have demonstrated their superior performance in mismatched conditions compared to state-of-the art discriminative models. However, this was investigated with a single database for training and another one for testing, which makes the results highly dependent on the particular databases. Moreover, recent developments from the image generation literature remain largely unexplored for speech enhancement. These include several design aspects of diffusion models, such as the noise schedule or the reverse sampler. In this work, we systematically assess the generalization performance of a diffusion-based speech enhancement model by using multiple speech, noise and \gls{brir} databases to simulate mismatched acoustic conditions. We also experiment with a noise schedule and a sampler that have not been applied to speech enhancement before. We show that the proposed system substantially benefits from using multiple databases for training, and achieves superior performance compared to state-of-the-art discriminative models in both matched and mismatched conditions. We also show that a Heun-based sampler achieves superior performance at a smaller computational cost compared to a sampler commonly used for speech enhancement.