Documents
Poster
DISTRIBUTED DIFFERENTIALLY-PRIVATE CANONICAL CORRELATION ANALYSIS
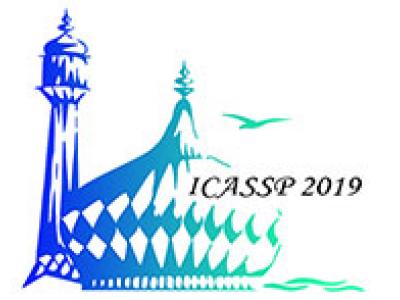
- Citation Author(s):
- Submitted by:
- Hafiz Imtiaz
- Last updated:
- 8 May 2019 - 10:10am
- Document Type:
- Poster
- Document Year:
- 2019
- Event:
- Presenters:
- Anand D. Sarwate
- Paper Code:
- Paper ID 3557
- Categories:
- Log in to post comments
We propose a distributed differentially-private canonical correlation analysis (CCA) algorithm to use on multi-view data. CCA finds a subspace for each view such that projecting the views onto these subspaces simultaneously reduces the dimension and maximizes correlation. In applications involving privacy-sensitive data, such as medical imaging, distributed privacy-preserving algorithms can let data holders maintain local control of their data while participating in joint computations with other data holders. Differential privacy is a framework for quantifying the privacy risk in such settings. However, conventional distributed differentially-private algorithms introduce more noise to guarantee a given level of privacy compared to their centralized counterparts. Our differentially-private CCA employs a noise-reduction strategy to achieve the same utility level as CCA on centralized data. Experiments on synthetic and real data show the benefit of our approach over conventional methods.