Documents
Poster
DISTRIBUTED ONLINE LEARNING WITH ADVERSARIAL PARTICIPANTS IN AN ADVERSARIAL ENVIRONMENT
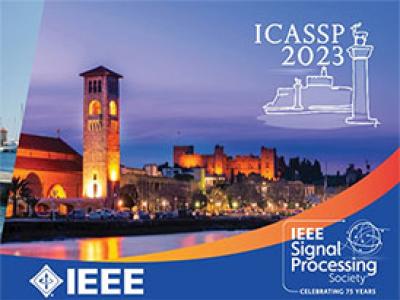
- Citation Author(s):
- Submitted by:
- Xingrong Dong
- Last updated:
- 22 May 2023 - 5:27am
- Document Type:
- Poster
- Document Year:
- 2023
- Event:
- Presenters:
- Xingrong Dong
- Paper Code:
- ICASSP-1869
- Categories:
- Log in to post comments
This paper studies distributed online learning under Byzantine attacks. Performance of an online learning algorithm is characterized by (adversarial) regret, and a sublinear bound is preferred. But we prove that, even with a class of state-of-the-art robust aggregation rules, in an adversarial environment and with Byzantine participants, distributed online gradient descent can only achieve a linear adversarial regret bound, which is tight. This is the inevitable consequence
of Byzantine attacks, even though we can control the constant of the linear adversarial regret to a reasonable level. Interestingly, when the environment is not fully adversarial so that the losses of the honest participants are i.i.d. (independent and identically distributed), we show that sublinear stochastic regret, in contrast to the aforementioned adversarial regret, is possible. We develop a Byzantine-robust distributed online gradient descent algorithm with momentum to attain such a sublinear stochastic regret bound.