Documents
Presentation Slides
Diversity in Fashion Recommendation Using Semantic Parsing
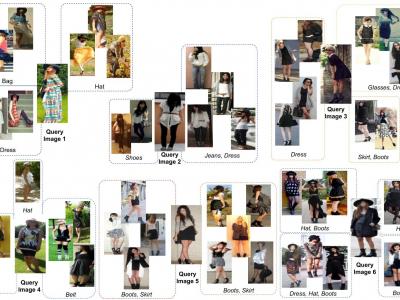
- Citation Author(s):
- Submitted by:
- Sagar Verma
- Last updated:
- 8 October 2018 - 4:26am
- Document Type:
- Presentation Slides
- Document Year:
- 2018
- Event:
- Presenters:
- Sagar Verma
- Paper Code:
- 2499
- Categories:
- Log in to post comments
Developing recommendation system for fashion images is challenging due to the inherent ambiguity associated with what criterion a user is looking at. Suggesting multiple images where each output image is similar to the query image on the basis of a different feature or part is one way to mitigate the problem. Existing works for fashion recommendation have used Siamese or Triplet network to learn features between a similar pair and a similar dissimilar triplet respectively. However, these methods do not provide basic information such as, how two clothing images are similar, or which parts present in the two images make them similar. In this paper, we propose to recommend images by explicitly learning and exploiting part based similarity. We propose a novel approach of learning discriminative features from weakly-supervised data by using visual attention over the parts and a texture encoding network. We show that the learned features surpass the state-of-the-art in retrieval task on DeepFashion dataset. We then use the proposed model to recommend fashion images having an explicit variation with respect to similarity of any of the parts.