Documents
Poster
DO DEEP-LEARNING SALIENCY MODELS REALLY MODEL SALIENCY?
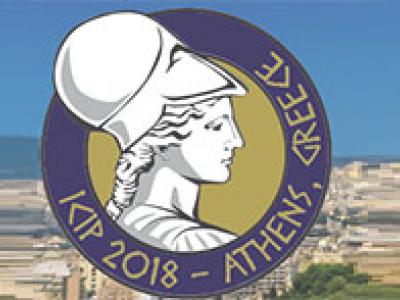
- Citation Author(s):
- Submitted by:
- Phutphalla Kong
- Last updated:
- 4 October 2018 - 9:19am
- Document Type:
- Poster
- Document Year:
- 2018
- Event:
- Presenters:
- Kong Phutphalla
- Paper Code:
- 1811
- Categories:
- Log in to post comments
Visual attention allows the human visual system to effectively deal with the huge flow of visual information acquired by the retina. Since the years 2000, the human visual system began to be modelled in computer vision to predict abnormal, rare and surprising data. Attention is a product of the continuous interaction between bottom-up (mainly feature-based) and top-down (mainly learning-based) information. Deep-learning (DNN) is now well established in visual attention modelling with very effective models. The goal of this paper is to investigate the importance of bottom-up versus top-down attention. First, we enrich with top-down information classical bottom-up models of attention. Then, the results are compared with DNN-based models. Our provocative question is: “do deep-learning saliency models really predict saliency or they simply detect interesting objects?”. We found that if DNN saliency models very accurately detect top-down features, they neglect a lot of bottom-up information which is surprising and rare, thus by definition difficult to learn.