Documents
Presentation Slides
Dual reverse attention networks for person re-identification
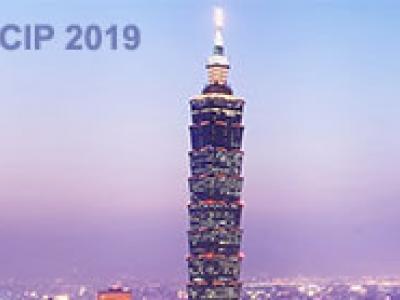
- Citation Author(s):
- Submitted by:
- Shuangwei Liu
- Last updated:
- 20 September 2019 - 11:04am
- Document Type:
- Presentation Slides
- Document Year:
- 2019
- Event:
- Presenters:
- Shuangwei Liu
- Paper Code:
- 1553
- Categories:
- Log in to post comments
In this paper, we enhance feature representation ability of person re-identification (Re-ID) by learning invariances to hard examples. Unlike previous works of hard examples mining and generating in image level, we propose a dual reverse attention network (DRANet) to generate hard examples in the convolutional feature space. Specifically, we use a classification branch of attention mechanism to model that ‘what’ in channel and ‘where’ in spatial dimensions are informative in the feature maps. Meanwhile, we introduce two branches of reverse attention modules in parallel way, which convert informative feature maps into hard examples of uninformative ones. In the proposed framework, both classification and dual reverse attention branches are learned in a joint way. Experimental results on three mainstream datasets demonstrate the efficacy of the proposed method.