Documents
Poster
AN END-TO-END MULTI-SCALE RESIDUAL RECONSTRUCTION NETWORK FOR IMAGE COMPRESSIVE SENSING
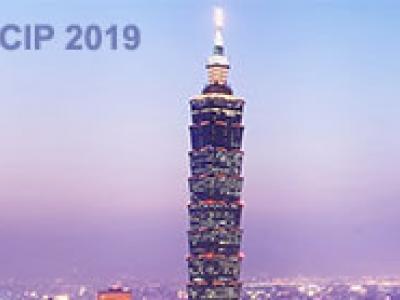
- Citation Author(s):
- Submitted by:
- Renhe Liu
- Last updated:
- 20 September 2019 - 8:10am
- Document Type:
- Poster
- Document Year:
- 2019
- Event:
- Paper Code:
- 2264
- Categories:
- Log in to post comments
Recently, deep-learning based reconstruction models have been proposed to improve recovery performance of compressive sensed image and overcome expensive time complexity drawbacks of iteration-based traditional algorithms. In this paper, we propose an end-to-end multi-scale residual convolutional neural network (CNN), dubbed MSRNet, to simulate image compressive sensing (CS) and inverse reconstruction process in real situation. In MSRNet, we apply three parallel channels with different convolution kernel sizes to exploit different-scale feature information. Besides, global and local residual architecture are also introduced to accelerate training process and enhance prediction accuracy of network. Moreover, different from generating CS measurements by random measurement matrix in previous methods, we integrate compressive sample process into MSRNet, which means measurement matrix can be learned by training the network. Experiments on benchmark datasets show our method outperforms other state-of-the-art algorithms by large margins and set a new level for CS reconstruction with competitive time complexity.