Documents
Poster
An Enhanced Hierarchical Extreme Learning Machine with Random Sparse Matrix Based Autoencoder
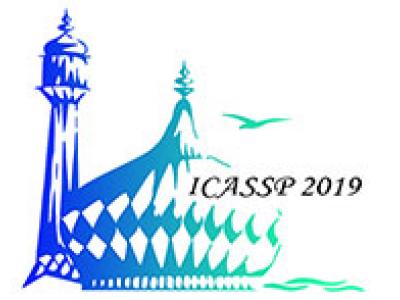
- Citation Author(s):
- Submitted by:
- Xiaoping Lai
- Last updated:
- 8 May 2019 - 10:06am
- Document Type:
- Poster
- Document Year:
- 2019
- Event:
- Presenters:
- Xiaoping Lai
- Categories:
- Log in to post comments
Recently, by employing the stacked extreme learning machine (ELM) based autoencoders (ELM-AE) and sparse AEs (SAE), multilayer ELM (ML-ELM) and hierarchical ELM (H-ELM) has been developed. Compared to the conventional stacked AEs, the ML-ELM and H-ELM usually achieve better generalization performance with a significantly reduced training time. However, the ℓ1-norm based SAE may suffer the overfitting problem and it is unable to provide analytical solution leading to long training time for big data. To alleviate these deficiencies, we propose an enhanced H-ELM (EH-ELM) with a novel random sparse matrix based AE (SMA) in this paper. The contributions are in two aspects, 1) utilizing the random sparse matrix, the sparse features can be obtained; 2) the proposed SMA can provide an analytical solution so that the high computational complexity issue in SAE can be addressed. Experimental results on benchmark datasets show that the proposed EH-ELM achieves a higher recognition rate and a faster training speed than H-ELM and ML-ELM.