Documents
Presentation Slides
ENHANCING END-TO-END MULTI-CHANNEL SPEECH SEPARATION VIA SPATIAL FEATURE LEARNING
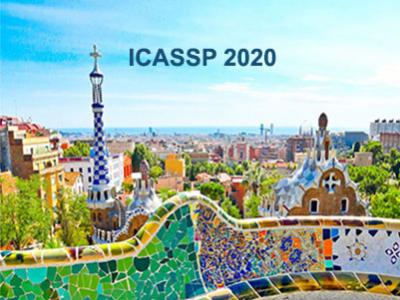
- Citation Author(s):
- Submitted by:
- Rongzhi Gu
- Last updated:
- 13 May 2020 - 10:45pm
- Document Type:
- Presentation Slides
- Document Year:
- 2020
- Event:
- Presenters:
- Rongzhi Gu
- Paper Code:
- SPE-P13.12
- Categories:
- Log in to post comments
Hand-crafted spatial features (e.g., inter-channel phase difference, IPD) play a fundamental role in recent deep learning based multi-channel speech separation (MCSS) methods. However, these manually designed spatial features are hard to incorporate into the end-to-end optimized MCSS framework. In this work, we propose an integrated architecture for learning spatial features directly from the multi-channel speech waveforms within an end-to-end speech separation framework. In this architecture, time-domain filters spanning signal channels are trained to perform adaptive spatial filtering. These filters are implemented by a 2d convolution (conv2d) layer and their parameters are optimized using a speech separation objective function in a purely data-driven fashion. Furthermore, inspired by the IPD formulation, we design a conv2d kernel to compute the inter-channel convolution differences (ICDs), which are expected to provide the spatial cues that help to distinguish the directional sources. Evaluation results on simulated multi-channel reverberant WSJ0 2-mix dataset demonstrate that our proposed ICD based MCSS model improves the overall signal-to-distortion ratio by 10.4% over the IPD based MCSS model.