Documents
Poster
ENHANCING MULTILINGUAL TTS WITH VOICE CONVERSION BASED DATA AUGMENTATION AND POSTERIOR EMBEDDING
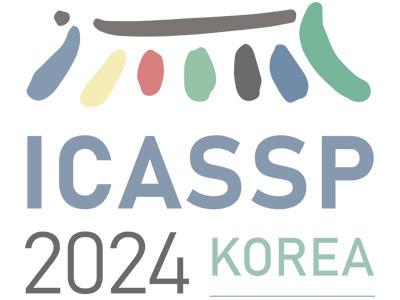
- DOI:
- 10.60864/71dj-0f94
- Citation Author(s):
- Submitted by:
- Hyun-Wook Yoon
- Last updated:
- 6 June 2024 - 10:21am
- Document Type:
- Poster
- Event:
- Presenters:
- Hyun-Wook Yoon
- Categories:
- Log in to post comments
This paper proposes a multilingual, multi-speaker (MM) TTS system by using a voice conversion (VC)-based data augmentation method. Creating an MM-TTS model is challenging, owing to the difficulties of collecting polyglot data from multiple speakers. To address this problem, we adopt a cross-lingual, multi-speaker VC model trained with multiple speakers’ monolingual databases. As this model effectively transfers acoustic attributes while retaining the content information, it is possible to generate each speaker’s polyglot corpora. Subsequently, we design the MM-TTS model with variational autoencoder (VAE)-based posterior embeddings. It is to be noted that incorporating VC-augmented polyglot corpora into the TTS training process might degrade synthetic quality, since the corpora sometimes contain unwanted artifacts. To mitigate this issue, the VAE is trained to capture the acoustic dissimilarity be- tween the recorded and VC-augmented datasets. Through the selective choice of the posterior embeddings obtained from the original recordings in the training set, the proposed model enables the generation of acoustically clearer voices.