Documents
Poster
ESA: Expert-and-Samples-Aware Incremental Learning under Longtail Distribution
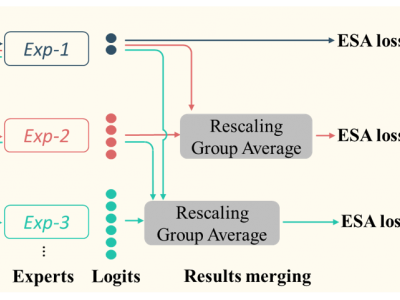
- Citation Author(s):
- Submitted by:
- Jie Mei
- Last updated:
- 6 April 2024 - 7:21pm
- Document Type:
- Poster
- Document Year:
- 2024
- Event:
- Presenters:
- Jie Mei
- Paper Code:
- MLSP-P29.5
- Categories:
- Log in to post comments
Most works in class incremental learning (CIL) assume disjoint sets of classes as tasks. Although a few works deal with overlapped sets of classes, they either assume a balanced data distribution or assume a mild imbalanced distribution. Instead, in this paper, we explore one of the understudied real-world CIL settings where (1) different tasks can share some classes but with new data samples, and (2) the training data of each task follows a long-tail distribution. We call this setting CIL-LT. We hypothesize that previously trained classification heads possess prototype knowledge of seen classes and thus could help learn the new model. Therefore, we propose a method with the multi-expert idea and a dynamic weighting technique to deal with the exacerbated forgetting introduced by the long-tail distribution. Experiments show that the proposed method effectively improves the accuracy in the CIL-LT setup on MNIST, CIFAR10, and CIFAR100. Code and data splits will be released.