Documents
Presentation Slides
ET: Explain to Train: Leveraging Explanations to Enhance the Training of A Multimodal Transformer
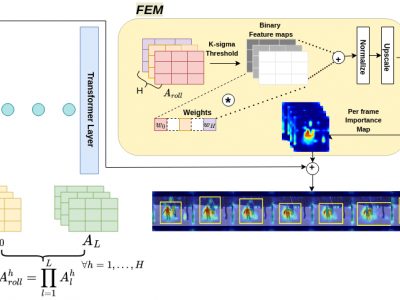
- DOI:
- 10.60864/c4gj-6283
- Citation Author(s):
- Submitted by:
- Meghna Ayyar
- Last updated:
- 12 November 2024 - 6:05am
- Document Type:
- Presentation Slides
- Document Year:
- 2024
- Event:
- Presenters:
- Meghna P Ayyar
- Paper Code:
- 1673
- Categories:
- Log in to post comments
Explainable Artificial Intelligence (XAI) has become increasingly vital for improving the transparency and reliability of neural network decisions. Transformer architectures have emerged as the state-of-the-art for various tasks across single modalities such as video, language, or signals, as well as for multimodal approaches. Although XAI methods for transformers are available, their potential impact during model training remains underexplored. Thus, we propose Explanation-guided Training (ET), leveraging an XAI method to identify salient input regions and guide the model to focus solely on these salient regions during training. We develop ET in a typical multimodal analysis framework using a multimodal transformer that operates on videos and signals. ET enhances the input by masking the non-salient regions for videos and enhances the signals with weights based on explanation scores for the sensor modality. Comparative evaluation with baseline vanilla training and the state-of-the-art XAI-based IFI method shows that ET consistently outperforms them. We benchmark our method on the publicly available UCF50 video dataset to demonstrate that ET is better than vanilla training and IFI. A risk detection corpus comprising egocentric videos and wearable sensor data is used for multimodal evaluation.