Documents
Poster
Extracting and Distilling Direction-adaptive Knowledge for Lightweight Object Ddetection in Remote Sensing Images
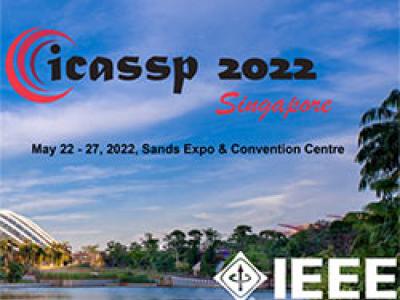
- Citation Author(s):
- Submitted by:
- Zhanchao Huang
- Last updated:
- 10 May 2022 - 12:10am
- Document Type:
- Poster
- Event:
- Presenters:
- Zhanchao Huang
- Paper Code:
- IVMSP-26.3
- Categories:
- Log in to post comments
Recently, some lightweight convolutional neural network (CNN) models have been proposed for airborne or spaceborne remote sensing object detection (RSOD) tasks. However, these lightweight detectors suffer from performance degradation due to the compromise of limited computing resources on embedded devices. In order to narrow this performance gap, a direction-adaptive knowledge extraction and distillation (DKED) method is proposed. Specifically, a dynamic directional convolution (DDC) is developed to extract the typical arbitrary-oriented features, and a direction-adaptive knowledge distillation (DKD) strategy is designed for guiding the lightweight model to learn the intrinsic knowledge of the RSOD task from the high-performance model. Experiments on public datasets demonstrate that the proposed method can effectively improve the performance of the lightweight RSOD model without additional inference costs.
Poster_Paper8521.pdf
