Documents
Poster
FACTORIZED HIDDEN VARIABILITY LEARNING FOR ADAPTATION OF SHORT DURATION LANGUAGE IDENTIFICATION MODELS
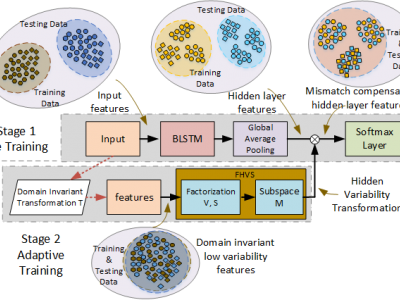
- Citation Author(s):
- Submitted by:
- Sarith Fernando
- Last updated:
- 12 April 2018 - 9:48pm
- Document Type:
- Poster
- Document Year:
- 2018
- Event:
- Presenters:
- Sarith Fernando
- Paper Code:
- SP-P4.7
- Categories:
- Log in to post comments
Bidirectional long short term memory (BLSTM) recurrent neural networks (RNNs) have recently outperformed other state-of-the-art approaches, such as i-vector and deep neural networks (DNNs) in automatic language identification (LID), particularly when testing with very short utterances (∼3s). Mismatches conditions between training and test data, e.g. speaker, channel, duration and environmental noise, are a major source of performance degradation for LID. A factorized hidden variability subspace (FHVS) learning technique is proposed for the adaptation of BLSTM RNNs to compensate for these types of mismatches in recording conditions. In the proposed approach, condition dependent parameters are estimated to adapt the hidden layer weights of the BLSTM in the FHVS. We evaluate FHVS on the AP17-OLR data set. Experimental results show that the FHVS method outperforms the standard BLSTM approach, achieving 27% relative improvements with utterance-level adaptation over the standard BLSTM for 1s duration utterances.
POSTER.pdf
