Documents
Presentation Slides
FANTOM: Federated Adversarial Network for Training Multi-sequence Magnetic Resonance Imaging in Semantic Segmentation
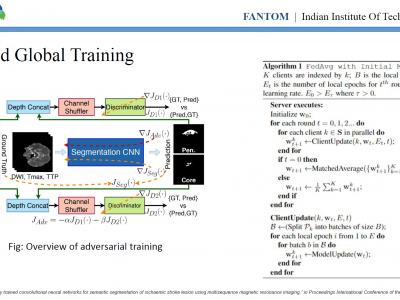
- Citation Author(s):
- Submitted by:
- Anupam Borthakur
- Last updated:
- 19 November 2024 - 7:20am
- Document Type:
- Presentation Slides
- Document Year:
- 2024
- Event:
- Presenters:
- Anupam Borthakur
- Paper Code:
- 2707
- Categories:
- Log in to post comments
Ischemic stroke lesions (ISL) segmentation aids clinicians in the diagnosis of stroke in acute care units. But, a generalized segmentation model requires data from various patients. However considering the data privacy, the patient's data is not available for centralized training. The Federated Learning (FL) framework overcomes this, but in FL, semantic segmentation is challenging due to the complex model, adversarial training, and non-independent and identically distributed dataset. In this work, we address these drawbacks for the segmentation of ISL into core and penumbra using multi-sequence magnetic resonance imaging data. Instead of applying cordinate-wise weight aggregation which is normally followed in vanilla FL aggregation strategies, we have applied the concept of neural matching in the proposed method named FANTOM. It helped in faster convergence of the training when performed with different non-IID data across clients. We have adversarially trained and tested our segmentation model comprises of generator and discriminator for the ISLES-2015 dataset. It is observed that keeping the discriminator model locally and aggregating only the generator model not only performs well but also lowers the communication burden in the FL framework.