Documents
Research Manuscript
FAST SINGLE-PERSON 2D HUMAN POSE ESTIMATION USING MULTI-TASK CONVOLUTIONAL NEURAL NETWORKS
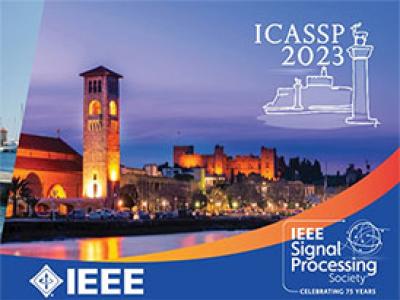
- Citation Author(s):
- Submitted by:
- Christos Papaio...
- Last updated:
- 31 May 2023 - 2:46am
- Document Type:
- Research Manuscript
- Document Year:
- 2023
- Event:
- Presenters:
- Christos Papaioannidis
- Categories:
- Log in to post comments
This paper presents a novel neural module for enhancing existing fast and lightweight 2D human pose estimation CNNs, in order to increase their accuracy. A baseline stem CNN is augmented by a collateral module, which is tasked to encode global spatial and semantic information and provide it to the stem network during inference. The latter one outputs the final 2D human pose estimations. Since global information encoding is an inherent subtask of 2D human pose estimation, this particular setup allows the stem network to better focus on the local details of the input image and on precisely localizing each body joint, thus increasing overall 2D human pose estimation accuracy. Furthermore, the collateral module is designed to be lightweight, adding negligible runtime computational cost, so that the unified architecture retains the fast execution property of the stem network. Evaluation of the proposed method on public 2D human pose estimation datasets shows that it increases the accuracy of different baseline stem CNNs, while outperforming all competing fast 2D human pose estimation methods.
2023041536.pdf
