Documents
Poster
Feature Dimensionality Reduction with Graph Embedding and Generalized Hamming Distance
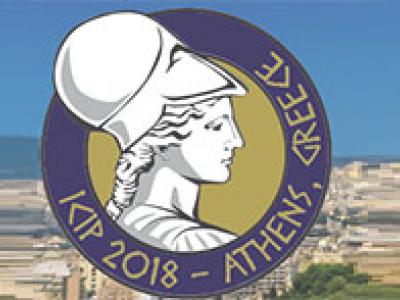
- Citation Author(s):
- Submitted by:
- Honglei Zhang
- Last updated:
- 7 October 2018 - 1:32am
- Document Type:
- Poster
- Document Year:
- 2018
- Event:
- Presenters:
- Honglei Zhang
- Paper Code:
- 3249
- Categories:
- Log in to post comments
Principal component analysis (PCA) and linear discriminant analysis (LDA) are the most well-known methods to reduce the dimensionality of feature vectors. However, both methods face challenges when used on multilabel data—each data point may be associated to multiple labels. PCA does not take advantage of label information thus the performance is sacrificed. LDA can exploit class information for multiclass data, but cannot be directly applied to multilabel problems. In this paper, we propose a novel dimensionality reduction method for multilabel data. We first introduce the generalized Hamming distance that measures the distance of two data points in the label space. Then the proposed distance is used in the graph embedding framework for feature dimension reduction. We verified the proposed method using three multilabel benchmark datasets and one large image dataset. The results show that the proposed feature dimensionality reduction method consistently outperforms PCA and other competing methods.