Documents
Presentation Slides
Feature Mixing-based Active Learning for Multi-label Text Classification
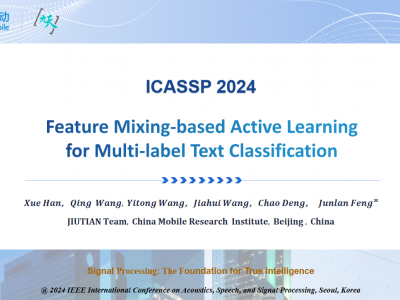
- DOI:
- 10.60864/9ptn-0k29
- Citation Author(s):
- Submitted by:
- Xue Han
- Last updated:
- 6 June 2024 - 10:32am
- Document Type:
- Presentation Slides
- Event:
- Presenters:
- Xue Han
- Paper Code:
- SLP-L11.3
- Categories:
- Keywords:
- Log in to post comments
Active learning (AL) aims to reduce labeling costs by selecting the most valuable samples to annotate from a set of unlabeled data. However, recognizing these samples is particularly challenging in multi-label text classification tasks due to the high dimensionality but sparseness of label spaces. Existing AL techniques either fail to sufficiently capture label correlations, resulting in label imbalance in the selected samples, or suffer significant computing costs when analyzing the informative potential of unlabeled samples across all labels. Facing these challenges, we propose an efficient two-stage sample acquisition strategy for multi-label active learning, called ALMuLa-mix. For saving the computational costs, ALMuLa-mix first attempts to identify unlabeled samples with novel features by employing a time-efficient feature-mixing method in conjunction with label correlations. Regarding the label imbalance, ALMuLa-mix then leverages the minority class in the labeled set to select a small batch of candidate unlabeled samples with greater inter-class diversity from the candidates with novel features. Experimental results on publicly available datasets show that ALMuLa-mix is superior to other strong baselines for handling multi-label text classification tasks.