Documents
Poster
Poster
Federated PAC-Bayesian Learning on Non-IID Data
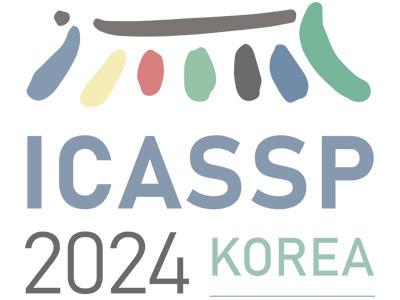
- DOI:
- 10.60864/1rxy-as96
- Citation Author(s):
- Submitted by:
- Zihao Zhao
- Last updated:
- 6 June 2024 - 10:32am
- Document Type:
- Poster
- Document Year:
- 2024
- Event:
- Presenters:
- Zihao Zhao
- Paper Code:
- MLSP-P10.5
- Categories:
- Log in to post comments
Existing research has either adapted the Probably Approximately Correct (PAC) Bayesian framework for federated learning (FL) or used information-theoretic PAC-Bayesian bounds while introducing their theorems, but few consider the non-IID challenges in FL. Our work presents the first non-vacuous federated PAC-Bayesian bound tailored for non-IID local data. This bound assumes unique prior knowledge for each client and variable aggregation weights. We also introduce an objective function and an innovative Gibbs-based algorithm for the optimization of the derived bound. The results are validated on real-world datasets.