Documents
Poster
Generalizing AUC Optimization to Multiclass Classification for Audio Segmentation With Limited Training Data
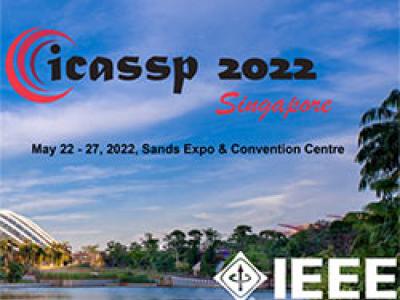
- Citation Author(s):
- Submitted by:
- Pablo Gimeno
- Last updated:
- 5 May 2022 - 4:19am
- Document Type:
- Poster
- Document Year:
- 2022
- Event:
- Presenters:
- Pablo Gimeno
- Paper Code:
- AUD-35.5
- Categories:
- Log in to post comments
Area under the ROC curve (AUC) optimisation techniques developed for neural networks have recently demonstrated their capabilities in different audio and speech related tasks. However, due to its intrinsic nature, AUC optimisation has focused only on binary tasks so far. In this paper, we introduce an extension to the AUC optimisation framework so that it can be easily applied to an arbitrary number of classes, aiming to overcome the issues derived from training data limitations in deep learning solutions. Building upon the multiclass definitions of the AUC metric found in the literature, we define two new training objectives using a one-versus-one and a one-versus-rest approach. In order to demonstrate its potential, we apply them in an audio segmentation task with limited training data that aims to differentiate 3 classes: foreground music, background music and no music. Experimental results show that our proposal can improve the performance of audio segmentation systems significantly compared to traditional training criteria such as cross entropy.